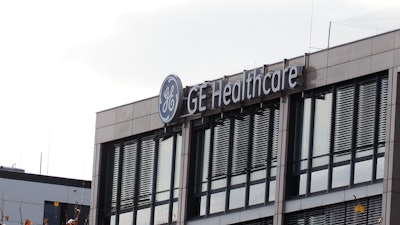
GE HealthCare today announced a collaboration with NVIDIA, expanding the existing relationship between the two companies to focus on autonomous imaging, beginning with autonomous X-ray technologies and autonomous applications within ultrasound.
GE HealthCare aims to develop AI-enabled X-ray and ultrasound systems by using the new NVIDIA Isaac for Healthcare platform, built on NVIDIA’s three computers used to build physical AI, including NVIDIA Omniverse for robotic simulation workflows. Using the NVIDIA Cosmos platform for synthetic data generation, physics-based sensor simulation, imitation, and reinforcement learning, GE HealthCare plans to train, test, and tune autonomous ultrasound and X-ray devices in a virtual environment before deployment in the physical world.
NVIDIA and GE HealthCare will initially focus on autonomous development within X-ray systems, specifically the potential utilization of the NVIDIA Isaac for Healthcare and Jetson platforms. GE HealthCare plans to explore Isaac for Healthcare platform and synthetic data generation to simulate various scenarios. This will help to automate repetitive tasks performed by a technologist in the patient exam room. The goal is to enable care teams to focus more of their time on direct patient care and complex cases. The companies will also explore the development of machine-to-patient interactions to autonomously lead the patient through the scan journey.
This work will build on a long-standing relationship between the two companies, spanning various areas of GE HealthCare’s business. As an example of previous synergies, GE HealthCare used NVIDIA technology for the development of GE HealthCare’s pioneering research foundation model SonoSAMTrack. SonoSAMTrack is a healthcare-specific research foundation model that GE HealthCare trained on approximately 200,000 image-mask pairs, which delineate diverse sets of regions of interest including organs and lesions, and exhibited an average similarity score of over 90%, indicating a high degree of segmentation accuracy.